Welcome to the fascinating world where data science meets finance! In today’s era of abundant data, the field of data science has revolutionized various industries, and finance is no exception. With the power of predictive modeling and risk analysis, data scientists are unlocking valuable insights that drive better financial decision-making. In this blog post, we’ll explore the intersection of data science and finance, diving into the realm of predictive modeling and risk analysis, and how they shape the financial landscape.
1. Understanding Predictive Modeling
Predictive modeling is like a crystal ball for finance. It involves leveraging historical data to create models that predict future outcomes. These models help financial institutions make informed decisions, ranging from credit scoring and default prediction to market and price movement forecasting. But before we jump into the fascinating applications, let’s understand the basics.
Predictive modeling comes in different flavors, each with its unique strengths. Regression models provide a solid foundation, uncovering relationships between variables. Time series models, on the other hand, capture patterns and trends over time. And let’s not forget the powerful Random Forests, a technique that combines the wisdom of many decision trees to make accurate predictions.
But wait, before we start making predictions, we need to prepare the data. Feature selection plays a crucial role in predictive modeling. Just like a chef selects the finest ingredients, a data scientist selects the most relevant features to ensure accurate predictions. It’s like choosing the perfect blend of spices to create a delectable dish.
2. Risk Analysis in Finance
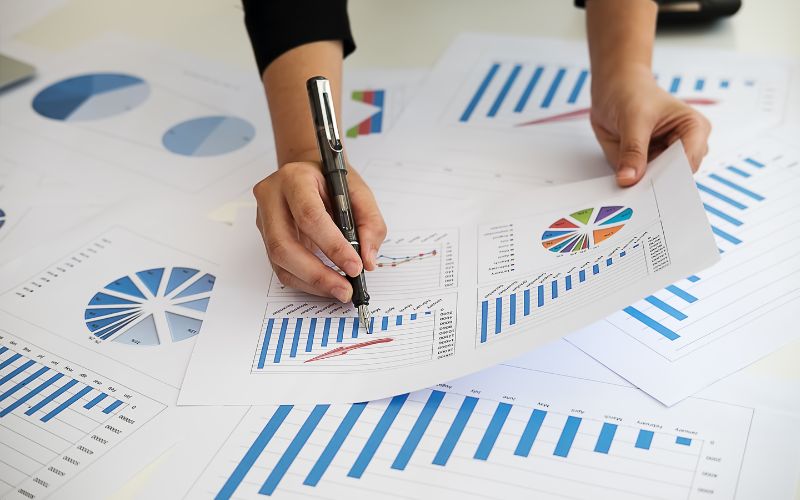
Finance and risk go hand in hand. Managing risks is essential for the stability and growth of financial institutions. This is where data science steps in with its arsenal of tools and techniques. Risk analysis involves assessing and quantifying various risks, such as credit risk, market risk, and operational risk.
To analyze risks effectively, data scientists employ sophisticated techniques. They dive into the vast sea of data, utilizing statistical methods, machine learning algorithms, and advanced modeling approaches. By understanding and quantifying risks, financial institutions can make informed decisions to protect their assets and investments.
3. Applications of Predictive Modeling and Risk Analysis in Finance
Now that we have grasped the concepts, let’s explore the exciting applications of predictive modeling and risk analysis in finance. Imagine a world where credit scoring is not a cumbersome process but a well-oiled machine. Predictive models can assess creditworthiness and predict default probabilities with impressive accuracy. This enables financial institutions to make informed lending decisions efficiently, making everyone’s life a little easier.
Fraud detection is another area where data science shines. With cleverly crafted models, anomalies and suspicious patterns can be identified, allowing for timely intervention and prevention. It’s like having a digital Sherlock Holmes uncovering financial misdeeds in real-time.
Portfolio optimization and asset allocation are key challenges in the investment world. Data science comes to the rescue with its predictive models that analyze historical data and market trends to suggest optimal investment strategies. It’s like having a personal financial advisor who can navigate the complexities of the market and guide you towards profitable decisions.
Market and price movements are often considered unpredictable, but data science begs to differ. By analyzing historical patterns and market indicators, predictive models can forecast future trends with a reasonable degree of accuracy. It’s like having a crystal ball that gives you a glimpse into the future of the financial markets.
4. Challenges and Considerations in Data Science for Finance
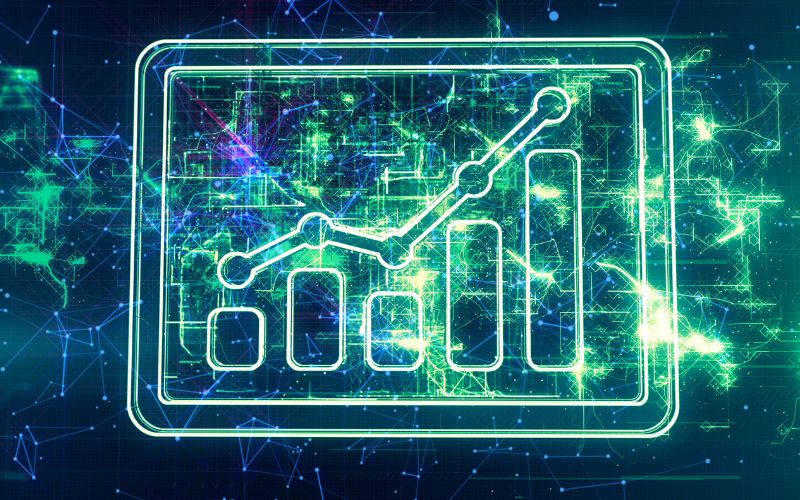
While data science holds immense promise, it’s not without its challenges. Ensuring data quality and implementing effective data governance practices are vital. After all, garbage in, garbage out. Data scientists need to carefully curate and preprocess the data to obtain reliable insights.
Handling imbalanced data and avoiding overfitting are also crucial considerations. Data scientists need to strike a balance, just like a tightrope walker, ensuring that the models are not overly biased or prone to errors. It’s like finding the perfect equilibrium between caution and confidence.
Interpretability and explainability of predictive models are essential in the finance industry. Stakeholders need to understand the underlying logic and reasoning behind the models’ predictions. Data scientists must strive to provide clear explanations, demystifying the complex algorithms. After all, transparency builds trust.
5. Case Studies and Success Stories
Let’s delve into some real-world examples that showcase the power of data science in finance. Take, for instance, a leading bank that successfully implemented predictive models to assess credit risk. By accurately predicting default probabilities, they were able to optimize their lending practices, resulting in reduced losses and increased profitability. It’s like turning a financial crisis into a golden opportunity.
Another success story revolves around a global insurance company that used data science techniques to combat fraudulent claims. By developing sophisticated fraud detection models, they saved millions of dollars, protecting both the company’s bottom line and the honest policyholders. It’s like being the hero in an action-packed financial thriller.
6. Future Trends and Innovations in Data Science for Finance
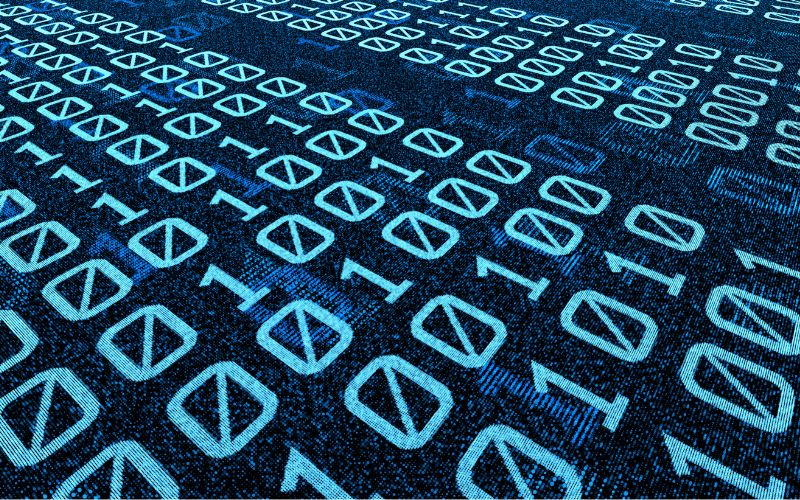
As technology advances, so does the field of data science in finance. Machine learning and artificial intelligence are continuously evolving, offering new opportunities to gain deeper insights and make more accurate predictions. Big data and alternative data sources are becoming mainstream, providing a wealth of information to fuel predictive models. Automated trading and algorithmic strategies are on the rise, streamlining financial operations and maximizing returns. The world of data-driven finance is on the cusp of transformation, and the possibilities are limitless.
7. Conclusion
Data science has emerged as a game-changer in the world of finance. Predictive modeling and risk analysis have revolutionized the way financial decisions are made, enabling institutions to navigate the complex landscape with confidence. From credit scoring to risk assessment, data science has unleashed a world of opportunities, driving innovation and efficiency. As we move forward, it is essential for financial professionals to embrace the power of data science and leverage its potential to unlock valuable insights. So, fasten your seatbelts and get ready to embark on a thrilling journey where data and finance intertwine, transforming the future of the financial world.
Remember, while the world of finance can be serious, a sprinkle of humor never hurts. After all, data science can be both informative and entertaining, just like a magician who reveals the secrets behind the tricks. So, embrace the power of data, put on your analytical hat, and get ready to witness the magic unfold in the realm of data science in finance.